C5 - Finding Flexibility in Large Flexible Loads: Making Demand Equivalent to Generation in Wholesale Markets
Authors
Debra LEW - Energy Systems Integration Group, USA
Richard O’NEILL - Consultant, USA
Erik ELA - Electric Power Research Institute, USA
Mark AHLSTROM - NextEra Energy Resources, USA

Summary
Increased levels of variable renewable energy will require more flexibility from all resources on the grid including generation, storage, and demand. At the same time, new loads may have significant power needs, which can have a large impact on the grid, but may also have some inherent flexibility in consumption, which can be beneficial to the grid. In fact, some of these new loads may depend on volatility in spot prices to be economically viable. Finally, automation, communications, and control are commercial at the customer level and can enable demand flexibility. While wholesale electricity markets in the US were designed with an expectation of an actively participating demand-side, the results have been disappointing. Customers have not been exposed to strong price signals or become accustomed to time-differentiated prices. In many places there are not good options for customers who want exposure to wholesale market prices.
In this paper, we discuss market participation of large loads in competitive wholesale markets. By participating in the day-ahead and real-time markets with bid-to-buy price curves, large loads can make markets more efficient, reduce overall system costs, reduce generation capacity needs, and serve their own needs efficiently. In this framework, demand acts equivalently to generation in the wholesale markets, and can provide energy and/or ancillary services, and set market clearing prices. Because demand is dispatched to set points, it provides reliability benefits to the system operator and can potentially reduce regulation reserve requirements. We discuss ERCOT experience with a load that is participating in markets in this way. Finally, we explore new loads such as electrified heat and transport, hydrogen production, and data centres, and their capabilities and economics in participating in this framework. We discuss the need for new market participation models that will prepare us for this future.
Keywords
Real-time pricing, bid-in demand, demand response, flexibility, wholesale electricity markets1. Introduction
Increased levels of wind and solar energy will require more flexibility from all resources on the grid including generation, storage, and demand. At the same time, new loads such as electrified heat and transport, hydrogen production, and data centres may have significant energy and power needs, which can have a large impact on the grid, but may also have some inherent flexibility in consumption that can be beneficial to the grid. Commercially available automation, communications, and control systems are available and can support demand flexibility. All this ushers in a new world of demand flexibility.
In wholesale markets, most load serving entities are price insensitive. That is, they are price takers. They may self-schedule a quantity of demand to purchase, but not a price associated with their willingness to consume different quantities. As such, they do not form wholesale prices or allow the market solution to more efficiently balance supply. Whether on the supply or demand side, self-scheduling can be economically disadvantageous to consumers and the self-scheduler because of inefficient operation and inefficient prices. Prices may rise higher than the value that consumers may get from electricity or may drop lower than the marginal costs of generators.
While wholesale electricity markets in the US were designed with an expectation of active demand participation, the amount of participation has been underwhelming in practice. The industry has been slow to expose customers to strong price signals. In modern society, electricity is essentially a basic need. Customers prefer simpler rate structures, and policymakers have wanted to shield customers, especially those who may be less equipped to manage their load, from time-differentiated or dynamic prices. Suitable, inexpensive automation, control and communication capabilities were largely absent. While flexibility did exist in loads, it may not have been seen as large an opportunity as the flexibility potentially inherent in modern data centres, hydrogen production, and electrification of buildings, industry, and transportation. Only recently have some regions made time-differentiated pricing default for all customers.
2. Shortcomings of demand response
To harness demand flexibility, demand reductions off a baseline usage have been packaged as supply-side resources such as demand response. While economists recognized the economic efficiency of demand being exposed to market prices and responding to shortages (high prices) and ultimately reducing capacity requirements, system operators may not feel confident in relying on customer behaviour to voluntarily reduce consumption. Traditional wholesale demand response programs treat demand flexibility on the supply side of the equation. Lack of confidence in price-responsiveness of demand affects both planning (should you build less generation, knowing that the demand may not respond to prices?) and operations (should you commit fewer units, knowing that demand may not respond?).
Demand response programs (and pricing options) are numerous and varied. Because demand response is treated as a supply-side resource, it suffers from the need for baselining, which can be easily manipulated, may drive perverse incentives, and can be difficult to do when demand response is called for multiple days in a row [1]. For example, every Christmas Eve, many industrial customers shut down for the holiday. In 2022 when winter storm Elliott coincided with the holiday, these industrial customers got paid for consuming below their baseline even though they were already planned to be shut down. Borenstein elegantly shows how using average prices results in higher capacity needs and less efficient capacity investment compared to using peak and off-peak prices; this same argument can be extended to finer timescales to demonstrate that real-time prices passed to customers will produce the most efficient capacity investment signals and result in the least capacity needs [1]. It then follows that average prices (or even peak and off-peak prices) plus demand response programs would likely require higher rates than would be needed for real-time pricing, because the utility needs to recover their revenue requirement.
In a low carbon future with high wind and solar, it is possible that electricity prices can alternate between zero when there is excess wind and solar that is being curtailed, and excessive high prices when net demand is high and shortage conditions trigger scarcity pricing. Figure 1 shows increasing levels of both low and high prices in a highly decarbonized system [2]. What is needed in this low carbon future is not simply ‘more supply’ or more demand response that curtails demand, but a shifting of demand to match supply, from high priced periods to low priced periods.
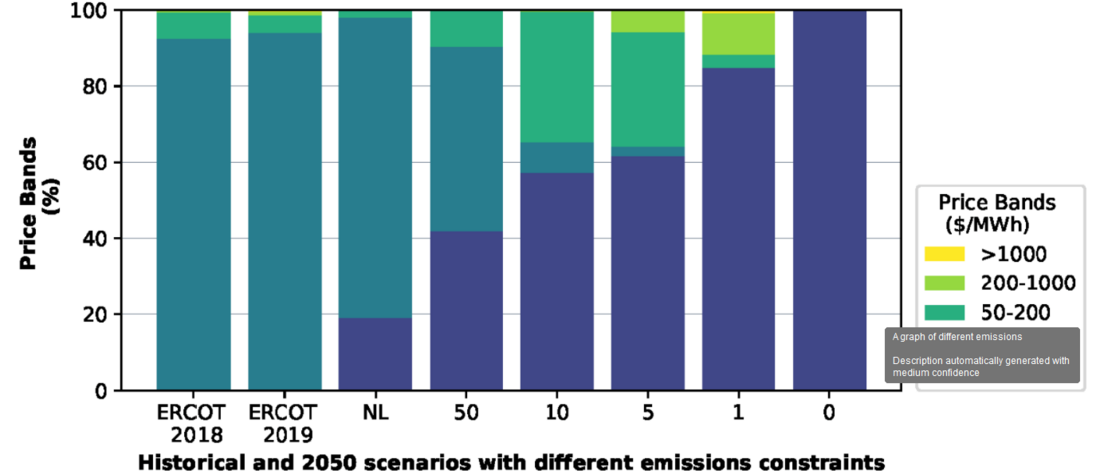
Figure 1 – Historical spot price bands for ERCOT in 2018-2019 and simulated spot price bands in 2050 with different emissions constraints in gCO2/kWh [2]
Source: C. Junge, 2022
2.1. Shortcomings of time-differentiated pricing options
Time-of-use pricing is a crude but simple option to shift demand. However, the grid is becoming more weather-dependent with higher levels of resources like wind and solar that vary with the weather and increasing loads from electrification of heating. Establishing peak, off-peak and shoulder periods a month or more in advance fails to reflect actual weather conditions and can lead to off-peak periods that coincide with high prices due to cold, cloudy or calm conditions. Wind, solar and load forecasts are more accurate closer to real time, so dynamic pricing that allows for price changes in day-ahead, intra-day, or real-time markets will align prices more closely with actual grid needs.
3. Exposure to prices – all the prices
During 2022, the Energy Systems Integration Group (ESIG) organized a task force to investigate retail pricing options to better align prices to grid needs and extract flexibility from demand [3]. The task force found that despite significant deployment of advanced metering infrastructure, demand flexibility underperformed its potential because, in general, most demand is not exposed to wholesale energy market price volatility [4]. Additionally, wholesale energy prices are only part of the customer’s total bill. Even though transmission charges, for example, may be easier to arbitrage against, most customers are not exposed to those either.
Kavulla makes the case that in the Electric Reliability Council of Texas (ERCOT), load management actions by customers to arbitrage 4 coincident-peak charges (4CP) can save customers more than arbitrage in the electricity markets [4]. 4CP is the mechanism by which transmission charges are allocated in ERCOT. A customer who is exposed to 4CP will have their transmission charge for the next year determined by their pro-rata share of the load during the peak 15-minute intervals in June, July, August, and September. Reducing their demand during these four peak periods can result in a significant bill savings for those customers. Voluntary reductions by customers who anticipate when they think the peak periods will occur can result in 3-5 GW of demand reductions [5]. This is evidence that there is flexibility in demand when customers are exposed to strong price signals, but this may not the most equitable or useful way to achieve it, as only about half of the load is allocated transmission charges in this way and the times of voluntary demand reduction are not guaranteed to coincide with the periods of greatest overall system benefit.
3.1. Real-time prices
There are various proposals for how demand can best respond to real-time prices. Some propose that large customers such as water pumping customers should have real-time tariff options. Some argue for “prices-to-devices” to allow smart devices and appliances to respond to prices autonomously.
There are also various definitions of real-time prices. Many proposals do not argue for the complexity and speed of 5-minute real-time wholesale market prices but rather hourly spot market prices such as Denmark uses. Some argue for day-ahead market prices which don’t have the volatility of real-time prices and can allow the customer time to manage their load for the following day. The retail provider Griddy passed real-time wholesale electricity prices directly to its customers. Unfortunately, their customers suffered severe financial hardship and Griddy went bankrupt after wholesale electricity prices were held at the $9000/MWh cap for several days during the Winter Storm Uri rolling outages in ERCOT.
4. Open-ended response to real-time prices
ISOs have the ability for demand (such as load-serving entities or other wholesale market participants) to submit price-sensitive bid curves detailing how much they are willing to pay for a quantity of electricity and their operating constraints. These resources are scheduled in the day-ahead market and can arbitrage off their day-ahead position in the real-time market if prices are higher (in which case they may want to reduce their consumption) or lower (in which case they may want to increase consumption).
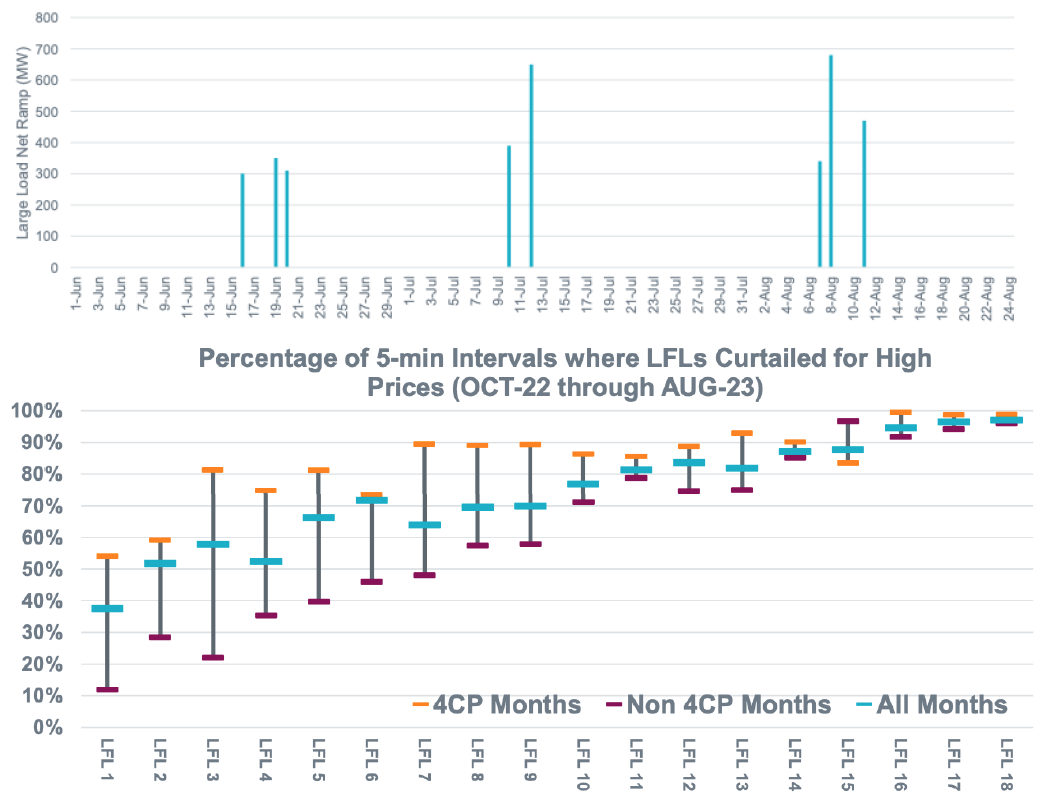
Figure 2 – Large Load Net Ramps within 15 minutes of energy prices above $250/MWh during June – August, 2023 (top) and Percentage of 5-minute intervals where large flexible loads (LFL) curtailed for high prices (bottom). The loads on the left have a significant difference in price-responsiveness during 4CP and non-4CP months, showing that they respond more to 4CP than to energy prices [6]
Source: Agee Springer, 2023
However, in practice, the first problem with open-ended response to real-time prices is that customer behaviour may not always appear to respond rationally to prices. In June-August of 2023, the top chart in Figure 2 shows reports eight days in ERCOT when over 300 MW of large loads ramped up within 15 minutes when prices were above $250/MWh [6]. They also report 27 days in 2023 where over 350MW of large loads remained online despite prices exceeding $500/MWh. Their analysis shows that many of these large loads may be responding more to 4CP than to energy prices (see Figure 2, bottom). This begs the question – should the system operator rely on responsiveness of these large loads to energy prices alone? Were they exposed to the real-time prices, or did other real-world considerations dominate their actions? Are real-time prices too myopic and failing to consider operational impacts on the resources or their other commercial considerations? How should the system operator commit its units? And if this were a system with a capacity market or obligation, how should planners consider responsiveness in determining capacity requirements?
Any “built-in” incentive that is external to the energy and ancillary services market can affect customer behaviour and make it difficult for the system operator to predict and to be willing to rely on customer behaviour. In addition to 4CP, incentives to charge storage or to produce hydrogen from onsite renewables rather than low-priced grid energy can also make it difficult for the system operator to predict behaviour. Today, system operators try to understand and anticipate customer behaviour as part of their load forecasting process in planning and operations. Tomorrow, customers may be more complex with various types of generation, storage, and flexible loads; they may be larger in size; and they may be numerous – this may result in too much complexity for system operators to manage in the way they do today.
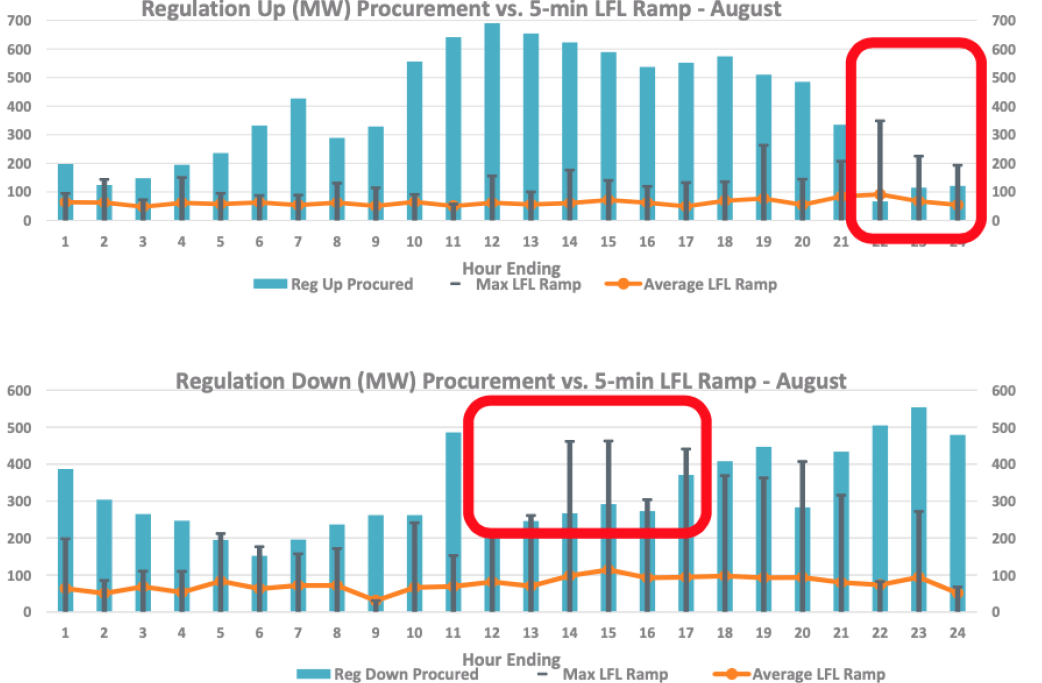
Figure 3 – Regulation up (top) and regulation down (bottom) shown in blue, is compared to up ramps (top) and down ramps (bottom) of large flexible loads. Average ramps are shown in orange and maximum ramps shown in grey whiskers. Circled in red are some maximum ramps of large flexible loads that exceed the regulation down reserves [7]
Source: Neel, 2023
The second problem with open-ended response to prices, such as the customer arbitraging off their day-ahead position or prices-to-devices, is that these actions only respond to price and not quantity. This can lead to an instability in supply and demand. Consider an example in which the marginal generator is very expensive and is dispatched to 50 MW, and the next-to-marginal unit is inexpensive. If 60 MW of demand responds to the high real-time price by turning down, the low price of the following interval may result in an increase in demand which may require the expensive generator again. This supply/demand instability could be a threat to system reliability. In the next section, we will show an alternative approach in which the ISO can efficiently dispatch quantities of demand based on prices in real-time and day-ahead markets.
The third problem with open-ended response to prices of large loads, aggregated loads, or a population of loads that act similarly (e.g., EV chargers that are set to the same price threshold or the same charging time) is that large, unmanaged changes in load can increase the need for regulation reserves (see Figure 3). Already in 2023, ERCOT has seen 155 five-minute intervals where the change in large load consumption exceeded the regulation reserves and 566 intervals where these changes in consumption were more than half the regulation reserves [7]. As a result of these types of impacts, ERCOT may need to increase regulation reserve requirements, which would increase system costs, or impose ramp rate restrictions on these large loads to mitigate their volatility.
Finally, the Griddy experience demonstrates how open-ended real-time prices require a type of customer that has the capability to monitor prices and adjust loads accordingly in real-time. If prices increase higher than they are willing to pay and they are unable to adjust their load in real time, they will end up paying that higher price. A different approach, to be explained next, allows customers to express their price-sensitivity and be dispatched by the system operator. This means that the customer cannot end up paying more than they are willing to pay.
5. Dispatching bid-in demand
Dispatch of demand in the real-time market is an alternative to open-ended response to real-time prices. In this framework, a customer submits a bid-to-buy price curve into the wholesale energy market. The market operator would clear supply against demand according to the price curves submitted and send a schedule of consumption to the customer that is generally aligned with the price of energy.
Overall system costs are lower because demand as demand is reduced when it would otherwise lead to high supply costs [8]. In addition, with demand bidding, the administratively determined scarcity price (e.g., set when there is insufficient reserve to meet requirements) will likely be triggered less, resulting in lower prices.
In this way, demand participates in the market in a manner that is equivalent to a generator. Demand can set the price, just as a generator can set the price. Operating constraints can be expressed through the bid which may include some firm load as well as different quantities of price-sensitive load at corresponding prices.
By dispatching demand, the system operator signals to the customer the quantity of demand that can be served at the price expressed by the customer’s bid. Because we are now controlling quantity on both the supply and demand side, this enhances and lowers the cost of system reliability. Depending on how much demand participated, it is possible that reserve requirements would decrease, as the system operator was able to adjust both sides of the supply/demand balance. Figure 4 shows a net load curve for a system with increasing levels of solar resources. On the right is an illustrative depiction of dispatched demand compared to generation and storage resources. Unlike demand response which only reduces demand, bid-in demand increases demand when prices are very low such as when there is solar oversupply.
Dispatching bid-in demand can overcome the problems mentioned in the previous section: system operators now know how the resource will act because the resource has expressed their price sensitivity in their bid; quantity is dispatched by the system operator so instabilities in supply/demand cannot occur; the system operator can ensure they ask their load resources to ramp at a level that is within their regulation reserves; and finally customers cannot pay more than they are willing to pay.
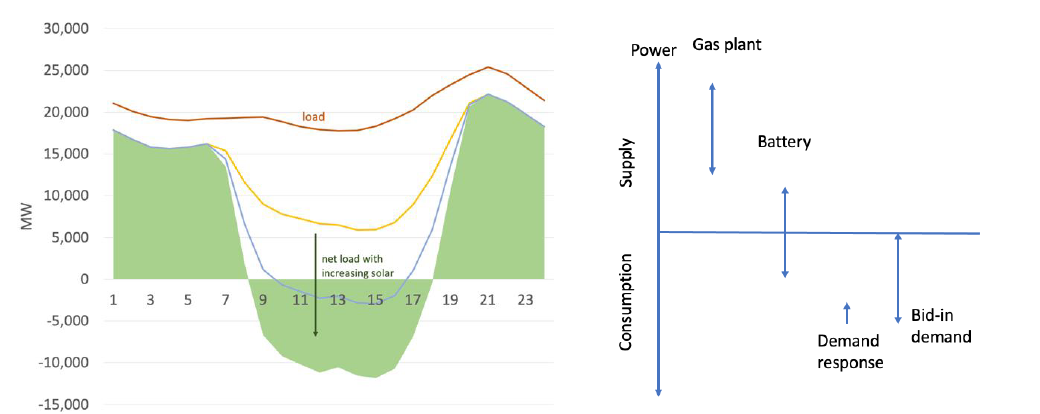
Figure 4 – Load and net load with increasing levels of solar (left). Illustrative depiction of how generation, storage, demand response and bid-in demand compare in helping to serve the needs of a future grid (right)
6. ERCOT’s Controllable Load Resource
Today, the only ISO in the US that allows this is ERCOT. ERCOT has three load resource participation models: First, load resources can provide ancillary services or energy in the form of demand response. For example, ERCOT is well-known for significant MW of load resources providing a ½ second responsive reserve to frequency deviations. Second, “Aggregate Load Resources” can aggregate resources smaller than 10 MW within one load zone and provide ancillary services or energy in the form of demand response. But we note that both are a form of demand response and treat load reductions as supply.
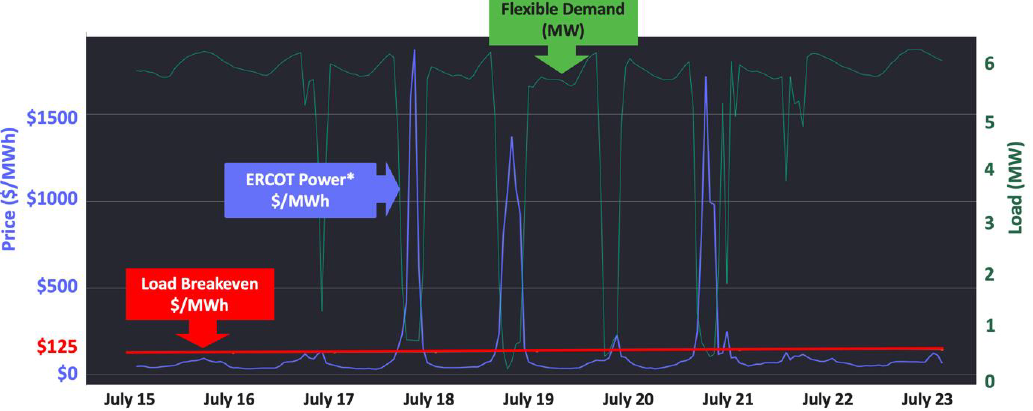
Figure 5 – Real-time Economic Dispatch of CLR. *ERCOT Load Zone West Real Time Settlement Point Price [9]
Source: A. Reimers, 2023
In contrast, Controllable Load Resources (CLRs) can control their load, both up and down, to respond to dispatch set points from ERCOT. In this way, CLRs act like a storage resource. The CLR model fits well to this dispatchable bid-in demand concept.
ERCOT requires CLRs to provide primary frequency response (PFR), just as they require this of wind, solar, and gas generators. This requirement may limit the loads that can or want to participate as CLRs because some loads may not have the capability to provide PFR (which is an autonomous response that can occur without any signal to the customer). Others, (e.g., ammonia synthesis) may have some components that can provide PFR (like electrolysis) and other components that cannot (like the synthesis of ammonia from hydrogen and nitrogen). While the PFR requirement is helpful for a small system like ERCOT where frequency is easily affected, it may not be as necessary for large systems and it may be a barrier to load participation, so system operators should give consideration as to whether it should be required. On the other hand, FERC Order 842 requires all new generators to be capable of PFR provision, so the PFR requirement is aligned with demand acting as generation in the wholesale market.
Today the only CLRs in ERCOT that are load-only, submitting bid-to-buy price curves, are cryptocurrency data centres operated by Lancium [9]. This type of load is a good fit for CLR for several reasons. First, there is a near linear relationship between electricity consumption and production of cryptocurrency. Approximately 95% of the data centre power needs is related to cryptocurrency production as can be seen in the Figure 5 when the cryptocurrency production is curtailed. Second, there is a clear breakeven threshold for the electricity consumption, making it straightforward to determine price-sensitivity. In Figure 5, the breakeven price is $125/MWh so the data centre curtails when the prices exceed that threshold.
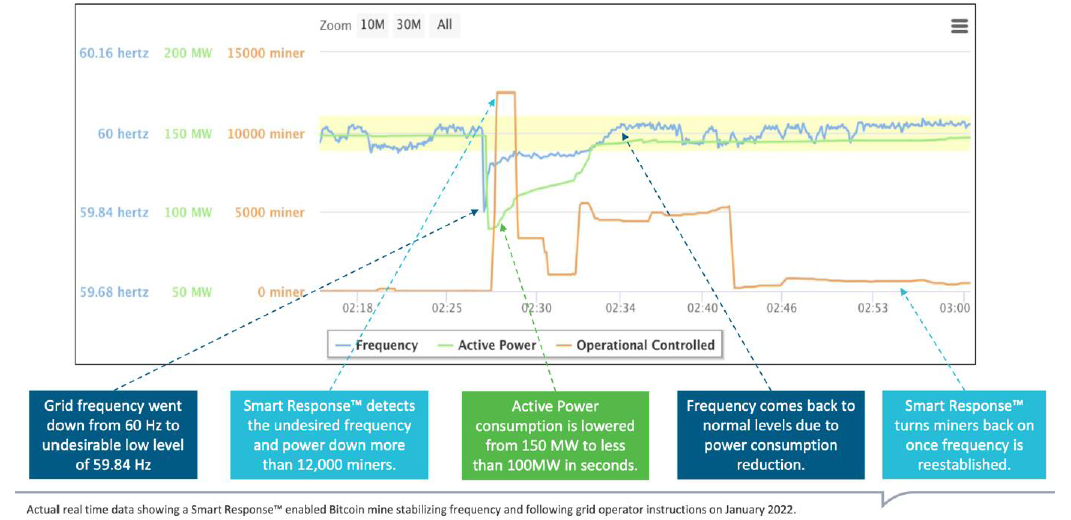
Figure 6 – CLR in ERCOT providing PFR [9]
Source: A. Reimers, 2023
Third, the load and its curtailment are easily automated and controllable. In fact, the automation and control are so fast that the data centres are able to provide PFR as shown in Figure 6.
7. Zonal vs locational pricing
In US markets, load is typically priced zonal while generators are priced nodal. A zone is a collection of nearby nodes, and the zonal price is a weighted average of nodal prices. Demand that responds to nodal prices (locational marginal prices – LMPs) while settling at zonal prices can potentially result in incorrect price signals. It is therefore important that market participation models allow loads to settle at their nodal rather than their zonal price. At this time, CLRs in ERCOT settle at zonal prices but proposed market changes would allow them to settle based on nodal prices.
8. Extreme Events
Extreme events are rare, but we note that during an extreme event like Winter Storm Uri, the risk of open-ended real-time prices becomes more of a price risk of high bills, whereas the risk of bid-in, dispatched demand to real-time prices becomes more of a quantity risk of not being able to buy electricity because the cost exceeds willingness-to-pay. With enough demand bidding, demand sets the scarcity price and the administratively determined (ORDC) scarcity price may never be needed.
9. New load types
In the future, we expect not only more cryptocurrency data centres but a plethora of other large new loads that may have flexibility. In addition to hydrogen, ammonia and other chemical products, a potential new flexible load is electrification of industrial heat for processes such as steel, chemicals, and cement. Resistance heating or heat pumps can be used in place of fossil fuel combustion. Because industrial processes can require significant heat, electricity requirements for thermal energy can be much higher than native electricity requirements for industrial processes. Variable renewables can produce electricity at extremely low cost per MWh but the output is variable. Because thermal storage, unlike electricity storage, is relatively inexpensive with costs that don’t scale linearly with duration, and has low losses, thermal storage provides a good match for variable renewable output.
Companies such as Rondo and RedoxBlox are now using renewable electricity to charge thermal storage for industrial processes. For example, a chemical refinery may have a 20 MW electric load and a 200 MW thermal load. To provide heat, they may build an oversized 700 MW of solar to charge the long-duration thermal storage that provides heat throughout the night (see Figure 7). However, on cloudy days they may be buying power from the grid to charge the storage. Because it only charges from the grid during low-priced hours, it does not increase resource adequacy requirements on the grid, as will be discussed later.
A typical industrial load may have a non-coincident demand charge and a time-of-use tariff. This is neither conducive to the plant economics nor to providing grid needs. For example, a peak consumption of this customer during times of oversupply, rather than times of grid stress, is helpful to the grid, but the non-coincident demand charge penalizes this behaviour. Time-of-use tariffs do not have the volatility of real-time prices so will not reflect the very low prices during times of oversupply, nor do they reflect real-time grid conditions, so the customer cannot align its load with grid needs.
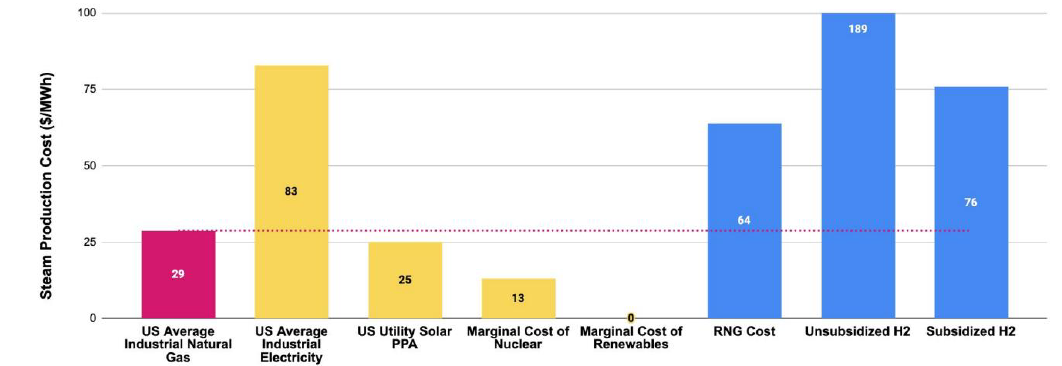
Figure 7 – Cost of producing steam as a function of energy source [11]
Source: M. Tuttman, 2023
Figure 7 shows that steam production from natural gas is cheaper than electrified heat based on average industrial electricity prices. However, steam production from new solar facilities is competitive with natural gas. Even better is steam production based on the marginal prices of nuclear or variable renewables. However, this requires exposure to real-time prices and the capability to control the load. Put another way, this customer is depending on price volatility to economically run their process.
However, this customer is not a simple load resource because of its generation and ability to export power, and many markets lack participant models for this type of resource. This hybrid load/generation may look more like a storage resource with its ability to produce or consume power (see Figure 8).
10. Market participation model for the future
This type of hybrid load/generation participant is likely to grow with the advent of hydrogen production facilities co-located with generation behind a point of interconnection. Larger loads are being announced with hydrogen and other fuel production facilities in the range of hundreds of MW to tens of GWs. For example, hydrogen production facilities in west Texas operating during the 70-80% lowest marginal priced hours can reportedly produce hydrogen for a levelized cost of $3/kg, which is equivalent to the hydrogen subsidy in the recent Inflation Reduction Act [10]. We expect an acceleration of co-located hydrogen production and renewable generation facilities.
ERCOT’s Private Use Network (PUN) is the only market participation model that we know of that can allow for this kind of hybrid load/generation resource. A PUN is an electric network connected to the transmission grid with both load and generation behind a point of interconnection, so that ERCOT does not meter the load directly but rather meters the net load and settles based on net load or net generation. During any interval, a PUN can act as a load or a generator providing energy and/or ancillary services. PUNs provide generation with a built in off-taker, provide load with a secure generation source, reduce peak demand, reduce exposure to 4CP transmission charges, mitigate exposure to transmission congestion, and can provide clean energy to a load. Traditionally, PUNs were industrial facilities like refineries, but today they include data centres and oil compressors that are connected to renewable generators.
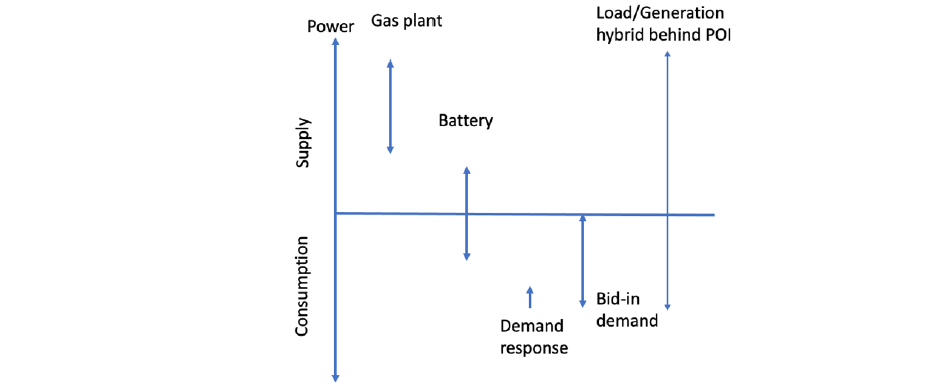
Figure 8 – Illustrative depiction of new hybrid load/generation resources that may be in our future
This hybrid load/generation resource may look like a large battery, but it may have asymmetric consumption and generation limits and may have more complex operating parameters than a battery. Today there are various modes of participation for batteries and who controls the state-of-charge of the battery [12]. With the complexity of this hybrid resource, ISO information of and control over the state-of-charge may be difficult. The most appropriate ways for these hybrid resources to participate are not clear yet. One option is that customers that control their own state-of-charge would be responsible for the feasibility of their submissions of operating parameters with enforcement via penalties for deviations from dispatch instructions, and potential grid disconnection for continued poor performance, much as generators have been treated.
11. Impact on interconnection
The market participation model may also affect interconnection. For example, a load/generation hybrid resource with 1 GW of hydrogen electrolysers and 3 GW of PV generation that wants to produce hydrogen 24/7 may never want to export more than 2 GW of excess electricity. Should this resource be studied in the interconnection process with 2 GW or 3 GW of export capability? If studied at 3 GW, there is the possibility that the resource triggers transmission upgrades that never get used. In another example, a cryptocurrency data centre that has a breakeven price of $125/MWh may never want to buy power when prices are high and the grid is congested. Should this resource be studied in the interconnection process during peak flow conditions with high congestion? Again, if studied during peak stress conditions, it may trigger transmission upgrades that are not used.
The interconnection impact is significant. Projects may be subject to high transmission upgrade costs, or they may be stuck in a multi-year interconnection queue backlogs. Figure 7 shows that industrial steam is very inexpensive based on marginal costs of renewables or nuclear resources. But it also shows that a utility-scale solar facility can provide steam cheaper than natural gas on average. Some projects today are considering utility-scale renewable energy facilities that are not grid connected but rather only serve that customer’s load (e.g. to charge thermal storage or to produce hydrogen). The number and diversity of grid resources, both on the supply and demand side, enhance system reliability, so it would be less efficient overall to have large facilities that are not grid-connected.
12. Impact on resource adequacy
Resource adequacy ensures sufficient resources to meet firm load at some point in the future under some level of reliability criteria (e.g., 1 day in 10 years). The future will likely see a growth in large loads including industrial heat, data centre, and hydrogen production that are either willing and able to curtail during high-priced intervals or are designed to capitalize on low-priced intervals. These loads need not necessarily incur a capacity obligation equivalent to their peak demand.
A customer who always bids in price curves below the market cap and who follows their dispatch signal (not consuming more than their set point during stress periods), will not be consuming during grid stress events where LMPs are at the market cap price, and therefore is not “firm load”. This customer may not impact resource adequacy and consideration should be given as to whether this customer should incur a resource adequacy obligation for that load. A system operator may not have confidence that this customer will follow their set point or may be concerned that this customer will self-schedule their load during a stress period. Therefore, there may be a need for penalties or possibly even curtailment or disconnection for customers who deviate from their set points during stress periods, or who bid in firm load.
Even the open-ended real-time price responsiveness discussed earlier has an impact on resource adequacy, although it is harder to quantify and may be harder for planners and operators to rely on. The ERCOT 4CP mentioned earlier is an example of open-ended responsiveness to critical peak periods and it has successfully helped to voluntarily reduce demand during peak periods of the four summer months [5].
13. Importance of Market Structure
One of the difficulties of bid-in demand is identifying the jurisdictions where this is possible. US competitive wholesale markets have the capability for load-serving entities or other wholesale customers to submit bid-to-buy curves in the day-ahead market, while few may do so. In the wholesale real-time markets, this option is not typically part of the current market design. Still, wholesale load serving entities may not have incentive to participate if there is not benefit to their customers that are being served. These customers that pay through the retail rates are not likely to be impacted by wholesale market prices that are dictating the consumption pattern of wholesale load. Regions where competitive wholesale markets line up with competitive retail markets (e.g., ERCOT, PJM, NYISO, ISONE) seem to be most promising to enable this, and as described above, ERCOT has already established the CLR participant model. The customer needs to be a qualifying scheduling entity in the market or to work with one to obtain access to the wholesale market.
In areas like SPP and MISO where there are competitive wholesale markets but not competitive retail markets, a resource would have to work through their local investor-owned utility, cooperative, or municipal utility to try to set up such an arrangement. A cooperative or municipal utility could have more flexibility in this regard than an investor-owned utility who would need to work with their regulator to set up a tariff that allows for real-time wholesale prices to be passed onto customers. But even so, a cooperative might not have access to the wholesale market and real-time spot prices since they in turn might be purchasing from a generation and transmission cooperative or investor-owned utility.
14. Conclusions
In the near future, we anticipate growth in new loads that have high power draw and some amount of flexibility. These include data centres, cryptocurrency, hydrogen production, and industrial heat electrification. We need market participation models for these new loads that incentivize them to align their demand with grid needs, as expressed by market prices. Without such market participation models, these new loads may lead to severe overbuilding of grid infrastructure, which leads to higher system costs. In addition, these loads may depend on some amount of low prices that require access to spot prices, or they may not be economically viable. Customers that submit bid-to-buy price curves rather than self-schedule their demand in wholesale markets help to reduce overall system costs, reduce loss-of-load expectation (depending on how price-responsive loads are considered in resource adequacy), and reduce generation capacity needs. If they participate in the real-time market and are dispatched to a set point, they provide added reliability benefits to the system.
It is critical to expose loads to price signals if we want them to provide flexibility. The closer the price signals are to real-time prices, the better signal they provide to reflect real-time grid needs. Exposing loads to real-time prices makes zero marginal prices an opportunity rather than a problem to be solved, as some in industry believe. Real-time pricing and bidding in demand are not for all customers. These are for customers who have automation, who understand their price sensitivity, who need the lower prices, and who have the capability to manage load. Customers who desire advance scheduling or don’t want as much volatility can always simply participate in the day-ahead market.
Because we anticipate increasing levels of more complex customers that have both generation and load behind a point of interconnection, these market participation models should consider how a customer may act as a net generator or net load at different times and participate in the market accordingly. There may be opportunities for industry to consider whether a universal participation model makes more sense than different participation models for different types of resources.
References
- S. Borenstein, M. Jaske, and A. Rosenfeld “Dynamic Pricing, Advanced Metering and Demand Response in Electricity Markets” (University of California Energy Institute, 2002)
- C. Junge, C. Wang, D. Mallapragada, H. Gruenspecht, H. Pfeifenberger, P. Joskow, and R. Schmalensee “Properties of Deeply Decarbonized Electric Power systems with Storage” (MIT, Feb. 2022 CEEPR WP 2022-003)
- D. Lew, E. Ela and C. Linvill “Aligning Retail Pricing and Grid Needs” (Energy Systems Integration Group, August 2023)
- T. Kavulla “Why is the Smart Grid So Dumb?” (Energy Systems Integration Group, Jan. 2023)
- ERCOT “2022 Annual Report of Demand Response in the ERCOT Region” (ERCOT, Dec. 2022)
- A. Springer “Integrating Large, Flexible Loads – Challenges for Grid Planning and Reliability” (Energy Systems Integration Group Fall Technical Workshop, Oct 25, 2023)
- E. Neel “Data Analyses on Large Loads” (ERCOT Large Flexible Load Task Force, Sep. 25, 2023)
- O’Neill, R., D. Lew, and E. Ela “Treating Demand Equivalent to Supply in Wholesale Markets: An Opportunity for Customer, Market, and Social Benefits” (Energy Systems Integration Group, May 2023)
- A. Reimers “Controllable Loads as a Resource” (Energy Systems Integration Group, Fall Technical Workshop, Oct 25, 2023)
- A. Reimers “Power-to-X: Concepts and Implications for Market Participants and Independent System Operators” (Energy Systems Integration Group, webinar, Oct 17, 2023)
- M. Tuttman “Thermal Energy Storage” (National Association of Regulatory Utility Commissioners, webinar, June 8, 2023)
- E. Ela and N. Singhal “Integrating Electric Storage Resources into Electricity Market Operations: Evaluation of State of Charge Management Options” (EPRI, Palo Alto, CA: 2019. 3002013868.)
